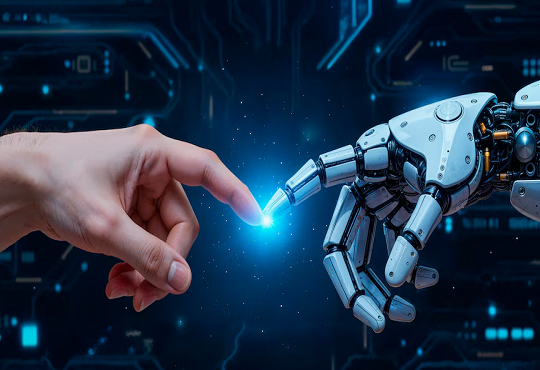
Data Management Strategies for AI-Driven Innovations in Banking
Dr. Parul Naib, Head - Data Science and AI, Reserve Bank Innovation Hub
Dr. Parul Naib, Head-Data Science and AI, Reserve Bank Innovation Hub, in an interaction with CIOTechOutlook, shares her views and thoughts on the main challenges in implementing real-time data pipelines for AI systems in banking as well as how data management strategies can be adapted to ensure seamless interoperability for AI-driven innovations.
Parul brings over 18 years of expertise in Machine Learning and Decision Science, leveraging her PhD in Public Health to lead the creation of data-driven systems and strategies that tackle complex developmental challenges across sectors.
Ensuring high-quality, accurate data is essential for AI systems to function effectively in the banking sector. How do organizations manage data integrity in large-scale AI-driven projects?
Before diving into implementation challenges, it is vital to align on the key Responsible AI principles that are essential for the ecosystem. These include fairness and Responsibility, Transparency & Explainability, Accountability, Privacy & Data Protection and Robustness & Security.
The use of ethical AI in finance is about balancing innovation with fairness, privacy, and accountability and these principles ensure that AI-driven financial services promote trust, equity, and security.
Ensuring the usage of high-quality veracious data is the foundation of effective and ethical AI. Yet, ensuring data integrity at scale remains a formidable challenge in banking, given fragmented legacy systems and heterogeneous data sources at banks. Organisations must ensure that data integrity is preserved through the adoption of Robust Data Governance Frameworks- establishing clear policies on data collection, storage, and usage aligned with RBI regulations, ensuring that these are compliance with customer data privacy and confidentiality laws such as the Digital Personal Data Act 2023.
Inaccurate or incomplete data does not just degrade model performance; it undermines customer trust and financial fairness.
Having multi-layered data integrity processes to safeguard data integrity is critical for organisations such as continuous data cleansing, validation, and lineage tracking to maintain consistency and auditability across diverse data sources. Leveraging automated data validation pipelines to check for missing values, duplicates, and anomalies at the time of data ingestion are essential to ensure its veracity and consistency. Further, implementing a secure Master Data Management (MDM) system enables a unified customer view across platforms ensuring a “single source of truth” for decision making and planning. At the same time having robust mechanisms for data lineage tracking ensures traceability and auditability at every step of data extraction, transformation and load (ETL) in line with regulatory compliance and auditability. Clear agreements for data sharing through secure API integrations, ensures that data exchanged between financial institutions and fintech partners occurs in a secure and confidential manner.
With the rapid expansion of AI use cases in banking, what challenges arise in scaling up data management processes to handle larger volumes of data while maintaining efficiency?
It is important to realise that scaling up is not just a technology or a storage issue - there are bigger concerns around governance, ownership and auditability. As more players handle sensitive data, robust access controls, encryption, and consent management are critical to prevent misuse and uphold ethical stewardship.
The key challenges include handling various data silos across departments- wherein different product lines and channels maintain their own “data marshes” which make it difficult to integrate all of it seamlessly in a data lake. Maintaining the data quality and consistency at scale continues to be a challenge.
The last few years have also seen an ever increasing volume, velocity and variety of the data which demands scalable infrastructure and efficient data processing frameworks. The introduction of real time UPI and IMPS has been a game changer in accelerating the exponential growth of digital transactions. At the same time, unstructured and multimodal data such as text, images, voices are increasingly being generated through various customer banking touchpoints, requiring the use of newer algorithms and faster computing capacity to process and interpret the data better.
Scaling up must also be in adherence to the regulator’s data privacy and security mandates, necessitating robust compliance and monitoring. Ultimately, scalable AI requires a combination of interoperable data architectures, regulatory alignment, and a data-first culture across the organisation.
Real-time data processing is crucial for many AI applications, such as fraud detection and customer service. What are the main challenges in implementing real-time data pipelines for AI systems in banking?
Real-time systems require more robust monitoring, security and controls to minimise the false positives and better and faster decision making. The successful utilisation of AI in banking often hinges on its real-time processing capabilities on massive data: detecting fraud in milliseconds, resolving customer queries instantly or the approving loans within minutes.
However, this real-time processing and decision-making introduces challenges around seamlessly integrating real-time data streams in legacy systems. Maintaining consistency across multiple data sources and systems in real-time and identification of data anomalies in live-streaming data and addressing them on the fly is critical especially if the data is being leveraged for AI based real time decisioning.
Deploying event-driven architectures and building API gateways capable of distributed parallel processing remain central to integrating real-time streams with core banking databases Edge AI can be used to run inference close to the source, helping detect latency spikes and model drift during deployment.
Additionally hybrid data architectures may be leveraged - with batch- mode data processing for certain features which update less frequently , and real-time processing for other features (like geolocation, transaction velocity) into online models at scoring time.
Ensuring low-latency data ingestion , parsing and inferencing at scale, while ensuring the model explainability remains central to the success of real time decision-making leveraging AI.
With the rise of open banking and collaboration with fintechs, how can data management strategies be adapted to ensure seamless interoperability for AI-driven innovations?
As open banking and embedded finance gain traction, banks and fintech have to navigate an increasingly complex data-sharing landscape. Seamless AI innovation demands interoperability across APIs, platforms, and regulatory frameworks. At the same time, this openness must be tempered with responsibility as unrestricted open data ecosystems risk unauthorised access, re- identification of PII data, or unintended secondary usage of data.
Having secure APIs and data platforms enable scalable, real-time data exchange while maintaining security. Adoption of API standards (OpenAPI, ISO 20022) to facilitate cross- institutional data exchange especially around suspicious actors. Establishing cross-industry data governance guidelines balancing innovation, privacy, and security are also essential elements of a robust strategy.
At the same time, leveraging federated learning models with multi-party computation enables collaborative AI without sharing raw data and addresses the security concerns. Ensuring robust protocols for anonymisation and tokenisation also helps to maintain customer data privacy and confidentiality. Establishing trust with customers and partners is central to open banking’s success-secure interoperability must go hand-in-hand with transparency.
Conclusion: A Vision for Responsible AI in Finance: Getting ready for the next wave
The future of finance is inclusive, intelligent, and ethically grounded. As AI becomes agentic and ubiquitous, there is an inherent need to design systems that empower every customer while protecting their rights and dignity. By integrating ethical safeguards alongside technological advances, we can ensure that access to credit is frictionless, banking is inclusive, and innovation serves the underprivileged.
CIO Viewpoint
National Technology Day 2025: Powering Progress...
By CIOTech Outlook Team
Aligning IT Roadmap with Business Objectives: A...
By Subhash singh Punjabi, CISO & Head Enterprise Architecture, Deepak Fertilisers & Petrochemicals Corporation Ltd
Empowering Women: Shaping the Future of Industry
By CIOTechOutlook Team
CXO Insights
GAZING INTO MY CRYSTAL BALL: Healthcare...
By Dr. Vishal Rajgarhia, Director, Finecure Pharmaceuticals Limited, Chairman, ASSOCHAM Pharma Council, Director, Ecuador India Cha
How Intelligent Swabs Saves Lives Post-Surgery
By Dr. Sreeram Srinivasan, CEO, Syrma Technology Private Limited
INTELLIGENT CONNECTED CARE: The Future Of...